Socrates UK 2016
Posted by Michael Okarimia in Conferences on August 17, 2016
I was fortunate to attend Socrates UK 2016 this year, which was hosted in the beautiful Wotton House, near Dorking in Surrey.
I’ve not attended a Socrates conference before but I’d heard great things about it, as Socrates has at heart the idea of promoting software craftmanship.
After arriving in the early evening and after hearty meal we were ushered into the main conference room for the evening Lightning Talks.
The two talks from that evening that stuck in my mind was one about Team smells, which has nothing to with hygiene but are signs that a team is not operating optimally. A rather informative mind map of Team smells was created and discussed
Franziska Sauerwein showed her presentation of Outside in TDD, something I’ve done throughout much of my career
As I met more and more attendees, I was struck by how friendly and welcoming they were to newcomers like myself. It was their desire to make software a craft rather than getting code out of the door as soon as possible, and attempting to improve professionalism of the industry.
Day One
Using the Unconference format, the agendas of the conference was unplanned before the conference start, where sessions were decided upon at the beginning of each day of the conference.
The on the first day of sessions I opted to attend the following:
- Anti-patterns anonymous
This was a session where the attendees arranged themselves to sit in a large circle and take turns to share their tales of software anti-patterns. It generally was a case of recounting war stories of incompetence and project failure, as we all took turns to tell our account of when a project didn’t go well, and then what we thought could have solved the problem.
Now I had an appetite for some technical sessions, so went on to attend a session about an application built on the SMACK platform
SMACK is an acronym listing the stack if technologies used in a single platform namely:
Spark, a distributed data processing framework, often used to analyse large datasets
Mesos, a distributed Linux kernel designed to operate on many nodes and host distributed systems
Akka, a Java runtime framework optimised for message based distributed systems
Cassandra, a highly scalable database
Kafka, a high throughput distributed messaging system
The application of this stack was used as a monitoring tool to log and analyse metrics from cloud based servers.
Having worked on a application at 7digital which uses Kafka I was interested to hear the problems that other session attendees had encountered and solved in their own platforms.
The next session I attended was titled Microservices vs Monoliths which became a discussion over the problems encountered with either architecture. I related my experiences of how breaking out a monolithic application into smaller APIs whilst sharing the same data store was not ideal, and how one had to consider fault tolerance when doing so.
Mashooq Badar of Codurance led a session on Serverless Architecture which I found very interesting. He talked about how his team built AWS Loft website was created only in AWS tech, namely using the Lambdas and API Gateway
One of the main advantages of using Lambda is the very low cost, rather than hosting your application inside EC2 or Beanstalk instances, one can pay for the resources used to execute the requests. Amazon will bill you appropriately per request, rather than for the resources used to maintain the uptime of your instances if they are not used.
After the hour was up, I moved onto a session titled Mentorship Patterns which is a role I’ve performed at 7digital, helping apprentices improved their skills and become more proficient software developers. I learnt that I could improve myself as a mentor by setting the what expectations I’d expect of the apprentice from the outset. Giving regular feedback was also essential, and this was something I’d do via regular 1-2-1’s.
As the end of the first day wound up, dinner was served, and in the evenings the lighting talks began. Attendees were encouraged to perform a short 5 minute talk in the evenings.
Domain Driven Development Strategic Patterns was a great talk by @Ouarzy whos blog is well worth reading
Radical Candor: Training guidance vs feedback. I wanted this talk to continue for more than its allotted five minutes as the concept of Radical Candor is to tell your team members constructively that they need to improve. The talker linked to this excellent article:
It sounds so simple to say that bosses need to tell employees when they’re screwing up. But it very rarely happens./
Forty Days of fixing, by @suzyhamilton commit to make a single change to a project to improve it, one change a day. Small changes to large messy project can slowly make it better, by following the boy scout rule; when making a change, always leave that part of code base in a better state than when arrived in it.
Discussion of the book Non-violent Communication
There was a story of introducing Agile into an enterprise waterfall project, which lead to a discussion of the book The Phoenix Project, a novel about how an IT project was turned around to save the company. It’s heavily inspired by the classic novel on the Theory Of Constraints by Eliyahu M. Goldratt, The Goal and has a contemporary setting.
Antony Marcano finished the lighting talks with by demoing how to applying SOLID principles to PageObjects when writing Acceptance Web Tests using Selenium & webdriver.
- Day Two
Serverless architecture was a subject the had piqued my interest so I spent a double session working on a hands on exercise
Mashooq Badar led a hands-on lambda session where we set up our own AWS web app powered by lambda. The lab was based upon his blog post on codurance’s site
When build applications on AWS it’s worth considering how to make them fit easily within the AWS ecosystem.
Amazon have created a guide to show how to do this
During the session I pushed my version of the lambda gateway application up up on my github account for future reference. This was probably my favourite technology session at SocratesUK.
Moving back onto the soft skills required to be a good developer, I headed over to join the session titled YOU’RE a developer?!
which was a discussion on the lack of diversity in technology sector, in particular the lack of women. There is still a cultural barrier that puts women off a industry sector which could do much more to become more professional. There is still a lot of sexism that goes unchallenged and we exchanged incidents of this occurring. Then we moved onto ideas around encouraging the changes in attitude that can help the situation. There’s clearly much more that can be done.
After another hearty dinner it was time again for the final five minute long Evening Lightning Talks
My former colleague Matt Butt spoke about the The Dangers of Empathy, and Emotional Contagion, and how to avoid Empathy Burn out. He recommended have a chat/slack room to vent one’s negative feelings. One should try to cultivate compassion without getting emotionally involved.
Finally Matthew Forrester demoed his code which could create diagrams of database schema from a YAML file
Socrates UK was the best conference I’ve attended. It really opened my eyes to the software craftmanship movement, some of the practices I was familiar with and use every day, but software craftmanship seems to tie them together succinctly. The attendees I met really seemed to care about writing great code that solves the right problem, and were a friendly and welcoming bunch to boot.
I want to attend next year’s conference and I wholeheartedly encourage others to do so as well.
- Further reading; information I learnt during the conference:
Tips for allow software developers to develop and grow. Seems to be informed by one of my favourite software books, Peopleware by Tom DeMarco and Tim Lister
Useful links:
Monitoring tool with SMACK architecture: instana.com
Secor is a tool to move Kafka logs into S3, created by pintrest
Software Craftsmanship Newsletter was created by @alebaffa
https://github.com/lscc/socrates-uk/wiki
RideLondon 2016
Posted by Michael Okarimia in charity on August 14, 2016
On 31 July I participated in the 2016 Prudential RideLondon cycling event, a closed road 100 mile course along the streets of London and the hills of Surrey.
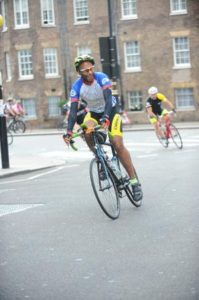
I completed the course in 4 hours 57 minutes, 53 minutes faster than my time last year so I was very happy with my improved performance.
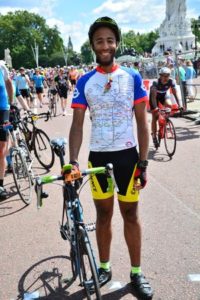
I set out to raise money for Haematology Cancer Care, a charity operating in UCL Hospital in Euston, London.
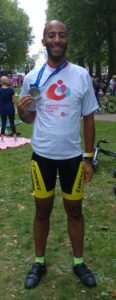
On my fund raiser page I raised more than £800 for Haematology Cancer Care
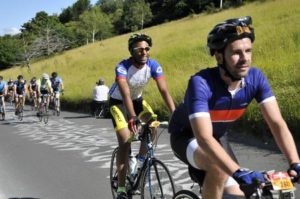
Boxhill
Here are my cycle stats with all the juicy ride data:
I couldn’t have done it without the great team of fellow cyclists working together in a mini peloton!
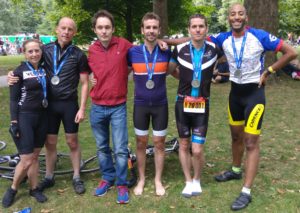
The Finishing Team!
Solving problems of scale using Kafka
Posted by Michael Okarimia in 7digital, API, Automation, Cloud, DevOps on March 1, 2016
At 7digital I was in a team which was tasked with solving a problem created by taking on a large client capable of pushing the 7digital API to its limits.  The client had many users and expected their numbers to exponentially increase. Whilst 7digital’s streaming infrastructure could scale very well, the requirement was that client wanted to send back the logs of the streams back to 7digital via the API. This log data would be proportional to the number of users. 7digital had no facility for logging said data being sent from a client, so this would be a new problem to solve.
We needed to build an Web API which exposed an endpoint for a 7digital client to send large amounts of JSON formatted data, and to generate periodic reports based on such data. The expected volume of data was thought to be much higher than what the infrastructure in the London data centre was capable of supporting. It was very slow, costly and difficult to scale up the London data centre to meet the traffic requirements. It was deemed that building the API in AWS and transporting the data back to the data centre asynchronously would be the best approach.
Kafka was to be used to decouple the AWS hosted web service accepting incoming data from the London database storing it. Kafka was used as a message bus to transfer the data from an AWS region back to the London data centre. It was already operational with the 7digital platform at this time for non real time reporting purposes.
Since there was no need to use the London data centre, there was no advantage in writing another application in C# that could be hosted on the existing Windows webservers running IIS. Given the much faster boot times of Linux EC2 instances and the greater ease of using Docker in Linux, we elected to write the web application in Python. We could use Docker to speed up development.
The application used the Flask web framework. This was deployed in an AWS ECS cluster, along with a nGinx container to proxy requests to the python API container and an DataDog container which was used for monitoring the application
The API was very simple, once the inbound POST request was validated, the application would write the JSON to a topic on a kafka cluster. This topic was later consumed and written into a relational database so reports could be generated from it. The decoupling of the POST requests from the process that that writes to the database meant we could avoid locking the database, by consuming the data from the topic at a rate that was sustainable for the database.
Since reports were only generated once a day, covering the data received during the previous day, there could be a backlog of data on the kafka topic; there was no requirement for Near Real time Data.
In line with the usual techniques of software development at 7digital, we used tests to drive the design of this feature and were able to achieve continuous delivery. By creating the build pipeline early on, we could build the product in small increments and deploy them frequently.
We had the ability to run a makefile on a developer machine which built a docker container running the Python web app. We could then use the Python test frame unittest to run unit, integration and smoke tests for our application. Â The integration tests were testing if the app could write to Kafka topics and the smoke tests were end to end tests, which ran after a deploy to UAT or Production to verify a successful deployment.
We successfully completed the project and the web application worked very well with the inbound traffic from the client. Since it was hosted in an EC2 cluster we could scale up both the number and the resources of the instances running our application. The database was able to cope with the import of the voluminous user data too. It served as a good example of how to develop an scalable web API which communicated with a database located in a datacentre. It was 7digital’s first application capable of doing so and remains in use today.